In the 1983 movie Trading Places, starring Dan Aykroyd and Eddie Murphy, two people from differing social strata involuntarily swap roles in a story played out within the context of financial markets. When the protagonists seize their opportunity to settle a score against two conniving broker brothers, they do so on the bustling futures trading floor amid a crowd of traders in shouting, speculative frenzy. There are several reasons to dismiss Trading Places as being “so ’80s.” One of these is that present-day financial markets are markedly different from the trading scenes portrayed in the film. While human traders used to inhabit the “engine room” of financial markets, that space today is occupied by engines alone. During the past 10-15 years, fully automated computer algorithms have made considerable inroads into the practices of financial markets, so much so that in several markets these algorithms—not human traders—are behind the bulk of orders being placed.
Financial algorithms typically work by sending orders to the market in response to particular preset conditions. They do so without direct human involvement and can operate within a fraction of a second. This automation does not mean that human beings have been eliminated fully from financial markets. Humans still develop the algorithms. Humans test, refine, and monitor the algorithms. Obviously, humans also take home the profit and losses generated by the algorithms. But the actual trading act, including the interactions between the automated algorithms themselves, is increasingly in the hands of trading machines.
The automation of financial markets has affected the chokepoints that exist within them. In the previously dominant inter-human market configuration, market panic of the sort portrayed in Trading Places created a particular type of chokepoint. Specifically, to be efficient, financial markets depend on a steady flow of useful, reliable information (as contrasted with distracting noise or misleading information). But this flow of information was blocked when human traders were caught in a seemingly irrational frenzy of trading. In other words, once emotional excitement began to replace cool-headed calculations, this led to an informational chokepoint—a situation in which noise effectively blocked the circulation of information.
While the automation of markets gives rise to new forms of chokepoints—some of which are installed intentionally as fail-safe measures and others, such as the liquidity chokepoints, emerge through more contingent factors—these often revolve around the same difference between information and noise. Furthermore, this difference and its manifestation in algorithmic finance tends to be related to a concern with market temperature—and “cool algorithms” may not always serve as an antidote to “overheated” markets.
Flash Crash Events
In financial markets, automation is often legitimized by a claim that it replaces a more volatile market climate—in which emotional human traders are prone to panic and herd behavior—with a system dominated by cool, forever-rational machines. However, the influx of computerized trading may not bring down the temperatures of financial markets. Much like the physical climate, the financial ecosystem is characterized by such frantic activity that overheating at times seems inevitable. In 2000, the U.S. securities market witnessed “on average about 5 million trades and quotes per day; in the fall of 2012, at peak times there were up to 5 million trades and quotes per second” (Malinova, Park, and Riordan 2013:1, original emphasis). This colossal growth is due to automated trading.
Skeptics of automated trading often point to the so-called Flash Crash of May 6, 2010, as an illustration of overheated markets. In this event, fully automated algorithms repeatedly bought and sold contracts to one another at high speed, a case of “hot potato” trading (CFTC-SEC 2010:15). This high-speed trading resulted in about one trillion dollars evaporating in just 30 minutes, with the majority of the losses taking place in less than five minutes. No less important, however, is that the algorithms also quickly restored the market almost to its pre-crash level.
In spite of this rapid recovery, the downward spiral of the Flash Crash gave rise to a rampant liquidity chokepoint: as prices rapidly dropped, many market participants—including many algorithms that usually provide liquidity—quickly left the scene. As a result, market circulation was brought to a halt. In the dramatic rendition of a former algorithmic trader who followed the events, “the market simply disappeared. For what felt like an eternity, but was more likely 30 seconds to a minute, there were no bids or offers displayed in the market for major stocks,” i.e., no buy or sell interest (Lauer 2012:2).
A halt in trading that lasts less than a minute may seem no real problem, certainly not a crisis. However, given the immense activity that can take place on a sub-second level in algorithmic markets, a void lasting up to 60 seconds can seem very long. More importantly, the liquidity chokepoint generated by the Flash Crash differs from the human-triggered informational chokepoints earlier described. The Flash Crash was not merely a matter of the flow of information being suspended in markets as emotions took over; rather, it was a matter of market participants withdrawing altogether. In other words, the market did not merely cease to exist as a place for information circulation because it was overloaded by noise. It ceased to exist because the players left the field.
Both regulators and market participants are keenly aware of the problems that might arise if trading algorithms collectively run for the exit. On the regulatory side, “circuit breakers” now can be activated to cool off overheated, excessively volatile markets. One set of these circuit breakers, the “limit up-limit down” mechanism, suspends trading if the price of an individual security moves beyond a particular range, which is calculated as a certain percentage deviation from the average price during the preceding five minutes of trading. Another set of circuit breakers takes a “market-wide” outlook. These are triggered by situations in which excessive volatility in one market may spill over into others and drain markets collectively for liquidity.
Meddling with the Flow of Information
Circuit breakers may be a helpful tool to control trading when the flow of orders accelerates. In the May 2010 Flash Crash, the downward spiral reversed in the opposite direction only after a brief market suspension was activated—as if the algorithms that continued trading could escape from their panicking collapse only after the plug had been pulled on trading and, after an interval, restarted. However, there are other types of chokepoints that cannot be alleviated by these kinds of circuit breakers. For example, a shady strategy in algorithmic trading consists of placing a large number of orders and then quickly cancelling them again before they are filled. The intended effect is to signal a strong market interest in a particular product and thereby lure other market participants into behaviors they would not otherwise contemplate.
To illustrate, suppose a trading algorithm has been activated to purchase 100 shares in a particular company and the buyer wants to sell them at the best possible price. By placing a large number of “bid” (buy) orders in the market, the algorithm sends a signal that demand is increasing and that the price should follow suit. By creating hype for the company via the “bid” orders, the selling price would be higher than could be obtained otherwise. After selling the shares, the algorithm then instantaneously would cancel the same “bid” orders. This algorithmic behavior can be carried out at extreme speed, in a fraction of a second. Since it can be difficult for regulators to determine whether the orders placed were intended to be filled or merely served as a smokescreen, it can be just as difficult to devise adequate regulation against such misrepresentation. Nevertheless, such strategies effectively block the circulation of “pure,” reliable market information; they introduce into the information flow a lot of noise, which can be difficult to discern. To bring this noise down to a reasonable level, many trading venues have introduced so-called “order-to-cancel ratios,” which stipulate how many orders may be cancelled per orders actually filled.
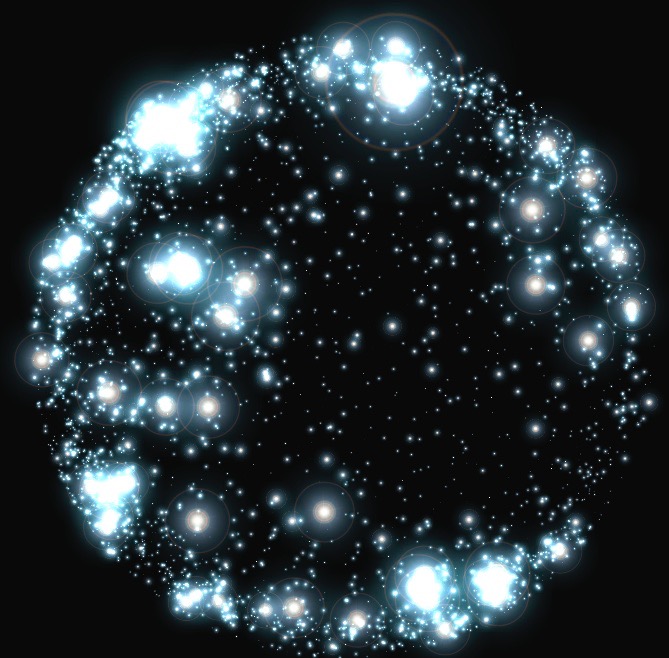
Figure 2: The First Stars in The Planetarium by Lise Autogena and Joshua Portway. Black Shoals; Dark Matter is a digital planetarium visualising the financial markets in real time. Stars in the planetarium represent companies on the world markets that move into constellations and galaxies depending on the movements on the markets. Trades happening on the stars produce light which provides food for an ecology of artificial life creatures that live and evolve within the starry sky link. Lise Autogena and Joshua Portway.
Material Chokepoints
There are other ways in which chokepoints might be created intentionally. In an interview conducted by Ann-Christina Lange of Copenhagen Business School, a former CTO of a large firm specializing in high-speed algorithmic trading explained that he occasionally would pull the plug on trading machines (Borch, Hansen, and Lange 2015). This was done not to avoid particular algorithmic trading patterns but rather to assess how the human staff coped with technical breakdowns. This type of intra-firm experiment addresses a material level of present-day financial markets, which looks very different from the inter-human trading floors depicted in Trading Places.
Most notably, a veritable technological arms race has haunted algo-financial markets for a long time now, the aim of which has been to shave off crucial milliseconds in the transmission of financial data. The most famous example is the connection between the exchanges in Chicago and New York (or rather between their data centers). Utilizing a set of increasingly faster infrastructures, from fiber-optic cables to microwave and laser transmission to millimeter wave links, a group of algorithmic trading firms is trying to position their algorithms into the “fast lane” ahead of competitors. While this strategy might prevent them from being caught in the kind of information congestion where everyone is “chasing the same signals” (Brown 2010), data transmission through microwaves or other channels is exposed to other types of chokepoints. For example, Donald MacKenzie (2017) describes how weather conditions can affect microwave transmissions. Meteorological phenomena such as rain, storms, even sunrise and sunset can bring this sort of data transmission to a halt. With the advent of algorithmic finance, the circulation of market information is therefore not merely exposed to a market-internal climate, in which chokepoints may emerge when markets overheat. Market information also circulates within a physical climate that might impinge negatively on the efficiency of ever-so cool algorithms.